The missing steps in the application of AI on remote health monitoring
Remote health monitoring refers to the use of technology to monitor a patient's health remotely outside of traditional healthcare settings, utilising connected devices like wearable sensors.
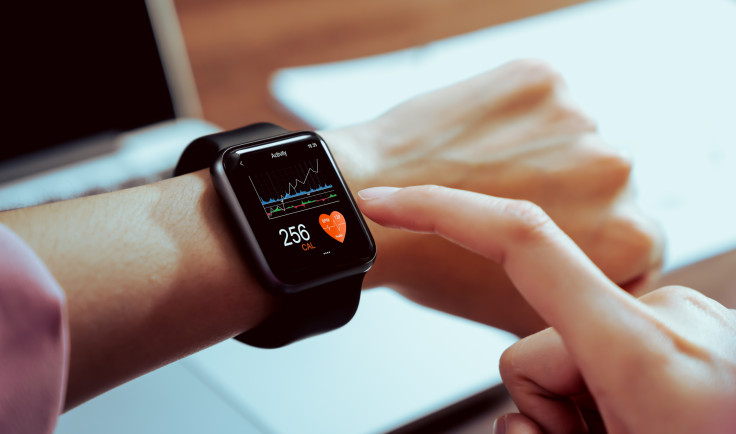
In 2019 Chris Mint, a healthy 30-year-old fitness enthusiast, had his life saved by an artificial intelligence algorithm running on his smartwatch. By processing the health-related parameters captured by the different sensors embedded in the device, the algorithm identified potential atrial fibrillation. After clinical exams, doctors diagnosed him with two leaking heart valves, a condition that could have caused a heart attack or stroke if not diagnosed on time.
Chris's story is not an isolated incident. Five similar cases resembling Chris's experience have been documented in recent years. These stories serve as compelling examples of the potential of Artificial Intelligence in remote health monitoring, showing its life-saving capabilities and the opportunities it presents for technological advancements and business growth.
According to a market analysis conducted in March 2022, the overall remote health monitoring market was valued at approximately 53.6 billion dollars in 2022, with projections indicating a potential value of around 175 billion dollars by 2027. A separate analysis by "Research and Market" in December 2022, specifically focusing on the AI application in Remote Patient Monitoring, estimated the value of AI in this market to be 1.4 billion dollars, with a projected growth of 4.3 billion dollars by 2027.
Despite the immense potential and widespread excitement surrounding AI technology, having worked in the field of AI applied to wearable technologies for several years, I have observed inherent challenges in the practical implementation of Artificial Intelligence in remote health monitoring. These challenges pertain to both efficiency and scalability. In this article, I want to outline these issues and offer potential solutions and suggestions. This practitioner's perspective will be valuable, particularly to health tech service providers and investors interested in this field.
A problem of definition
One of the first issues revolves around definitions. There is often confusion between remote health monitoring and eHealth, with even technical professionals considering them synonymous. This lack of differentiation leads to a failure in recognising the distinct applications of Artificial Intelligence in each domain.
Remote health monitoring refers to the use of technology to monitor a patient's health remotely outside of traditional healthcare settings, utilising connected devices like wearable sensors to collect and process real-time data on vital signs, physical activity and other relevant health metrics. On the other hand, eHealth considers a broader range of technologies in the healthcare field whose aim is to enhance medical services and information management.
These technologies are for example telemedicine, clinical decision-support services, advanced image diagnostic and, among others, remote health monitoring. In all these different areas, artificial intelligence can play a role in different ways. For instance, in some telemedicine platforms, AI-based virtual assistants and chatbots assist patients in therapy engagement and/or support doctors in complex diagnoses; differently in image diagnostics, AI-powered systems analyse medical images like X-rays, MRIs, accurately detecting abnormalities such as cancer, often surpassing human experts in accuracy.
It is essential to understand that each area of application is ontologically different from the others and presents unique challenges. Romans used to say, "Divide and conquer". Following this statement, in this article, we will focus solely on the issues concerning remote health monitoring.
Standardisation issues
On a deeper level, the second significant challenge lies in the lack of standardisation within the data-sharing pipeline. As said, Remote health monitoring involves the deployment of various devices and sensors to collect and transmit patient data from remote locations to healthcare providers.
However, sensor manufacturers often operate separately from the system integrators and without standardised protocols in most cases. This situation creates compatibility issues and makes it difficult to aggregate data from multiple sources. Consequently, this fragmented data hampers the ability to obtain a comprehensive and holistic understanding of a patient's health status and make also difficult to establish robust safeguards for sensitive patient information.
To better understand this issue, let's envision a hypothetical scenario where an AI algorithm is employed for the early identification of heart irregularities. By utilising a wearable sensor such as a pulse-oximeter, it becomes feasible to measure the user's heart rate throughout the day. However, it is important to recognise that heart rate is contingent upon the individual's physical activity level.
Therefore, in order to effectively accomplish the aforementioned objective, reliance solely on heart rate data is inadequate. The algorithm must also encompass an additional set of sensors to monitor the user's physical activity. If data from these various sensors is not accessible in its original form, the algorithm simply cannot function. A single stream of sensor data is often inadequate by itself, as it is the combination and aggregation of data that truly empowers the functionality of AI
Standardisation issues in remote health monitoring are closely tied to an unresolved trade-off between technology efficiency and data privacy. While rapid data processing and real-time monitoring enable early detection of health issues and prompt medical interventions, these approaches can conflict with stringent data privacy requirements.
Protecting sensitive health information may require additional security measures and data anonymisation techniques, introducing complexities that can impact the efficiency of AI-based health monitoring systems and also exacerbate biases stemming from inadequate datasets. Addressing this trade-off poses a significant consideration for policymakers in the future.
Technological challenges
So far we've focused only on higher-level standardisation and policy-related problems. In reality, there are also some technical challenges to tackle for the effective application of Artificial Intelligence in health monitoring.
The first technological challenge is the adoption of explainable AI models. Currently, many AI algorithms used in health monitoring, such as the ones detecting heart anomalies, rely on opaque "black box" models like deep neural networks. These models lack transparency and interpretability, making it challenging (sometimes impossible) to understand or explain their decisions. While this may be acceptable for certain use cases, it is not suitable for digital healthcare where the interpretability of the results, also among clinicians, constitutes a fundamental aspect.
The second technological challenge concerns the dependency on the final user. Successful remote health monitoring relies on user adherence to protocols. However, user compliance varies and incomplete or inaccurate data input can limit the effectiveness of the technology itself. To address this problem, systems should include self-check mechanisms to verify data reliability and device usage. For instance, in a case like a user wearing a smartwatch, algorithms should be implemented to ensure correct placement on the user's wrist.
In conclusion
In conclusion, we can say that businesses in the health tech sector must grasp and navigate these challenges if they want to fill the gap between theory and practice and make their products scalable. Resolving standardisation issues, balancing efficiency and privacy and adopting explainable AI models are vital for successfully implementing and commercialising AI-based remote health monitoring solutions.
Overcoming these obstacles is far from easy for a single company and it will probably require collaboration among the different stakeholders involved, tech-leading companies and policymakers as firsts. However, if you are an investor, you may want your health tech companies must have a strategy in place to address these challenges and meet the requirements. Otherwise, there is a risk that your technologies may not be profitable or, even worse, useful.

I am an ML&Sensor Fusion Engineer working in Cambridge, UK, for FocalPoint Positioning, a cutting-edge startup working in the field of navigation systems and motion tracking technologies.
During my career path, I collaborated with important companies and institutions in the Health-tech field like the Laboratory of Movement Analysis at EPFL, Lausanne, the laboratory of Brain-Computer Interface, the University of Tübingen, and health-tech startups in Italy & US.
Beyond my experience in the industry, I always coupled my career path with the academy. I am an external lecturer at the University of Padua for the faculty of "ICT for Internet and multimedia“ where I teach the course "Sensor Fusion for e-health" (an advanced version of the course I published on Youtube). I have a record of scientific and educational publications related to Machine Learning, Sensor Fusion, and general AI.
© Copyright IBTimes 2024. All rights reserved.