Here's how self-driving cars can detect dangerous roads using sound and AI
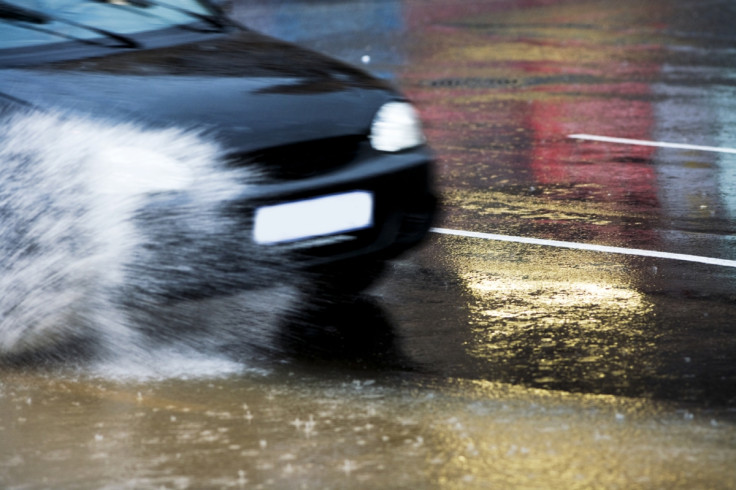
Researchers from the Institute of Electrical and Electronics Engineers (IEEE) have found a way to detect when roads are dangerously wet by using an artificially intelligent neural network of computers, which could help to make sure self-driving cars stay safe during bad weather.
According to the latest statistics from the US Department of Transportation, wet pavements caused 959,760 crashes and caused the deaths of 4,789 people over 10 years, between 2002 and 2012, making it the cause of 74% of all weather-related crashes in the US, and weather-related crashes make up 23% of all vehicle crashes in the country.
Engineers and scientists have been trying to come up with a system that can aid drivers in detecting where roads are likely to be the most dangerous.
IEEE's team decided to see if it would be possible to detect how slippery a road was by analysing audio feedback from the car's tyres, and to that end they used recurrent neural networks (RNN) – a type of artificially intelligent network of computers – and a shotgun microphone to monitor all the sounds the rear tyre on a 2014 Mercedes CLA produced when it came into contact with a road during different weather and at varying speeds around the Greater Boston area in Massachusetts.
The research is still in early stages, but initial tests showed an unweighted average recall (UAR) of 93.2% across all vehicle speeds, including when the car was at a standstill in the middle of traffic, because the microphone was still able to record audio from vehicles that went past. This is also the first time a deep learning approach of neural networks has been used to tackle road condition detection.
The difficulty in detecting bad road conditions
The IEEE is not the first to try to crack road surface conditions using sound. Another similar study by the Technical University of Madrid in 2014 used support vector machines (SVM) – a type of machine learning model – to analyse the sounds from the tyre meeting the road and classify the different sounds made by the asphalt.
However, the researchers found the range of surface types that could be predicted were limited, and unrelated audio input like the sound of pebbles bouncing against the tyres could create false predictions.
Also, in October 2012, researchers from the University of Toyama in Japan showed off a system that used surveillance cameras on cars to detect road surface conditions by looking at the road reflections highlighted by the headlights of other drivers' cars passing by, but the system requires other drivers to also be on the road, and video detection systems often find it to accurately detect road conditions in fog, snow and poor light.
"This method is shown to be robust to vehicle speed, road type and pavement quality on a dataset containing 785,826 bins of audio. It outperforms the state-of-the-art SVMs and achieves an outstanding performance on the road wetness detection task with an 93.2% UAR for all vehicle speeds and the more challenging speeds being those below 2.9mph, including vehicle stationary mode," the researchers wrote in their paper.
The study, Detecting Road Surface Wetness From Audio: A Deep Learning Approach, is published on Cornell University Library's open source database.
© Copyright IBTimes 2024. All rights reserved.