How MIT robots are helping to revolutionise hospital maternity wards
MIT CSAIL found that robots were much better at helping doctors and nurses to assign tasks than manual labour.
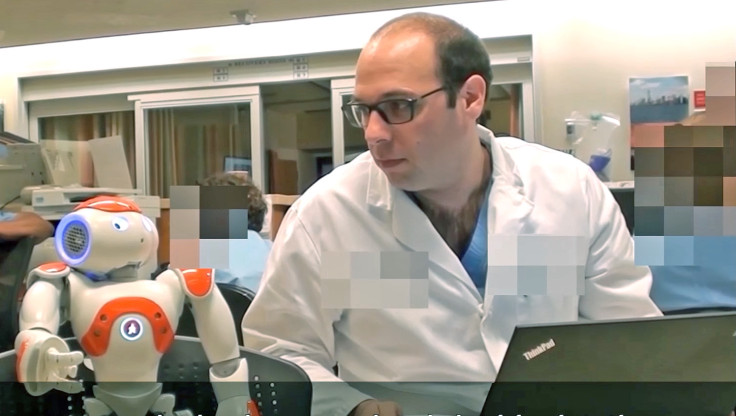
Computer scientists at MIT have found that while robots might not be capable of taking over our jobs any time soon, they would however be quite useful when it comes to helping humans with a fairly difficult challenge – prioritising tasks, allocating resources and time management.
Researchers from MIT's Computer Science and Artificial Intelligence Lab (CSAIL) have spent over two years looking at whether robots could be put to use to provide help to medical professionals in ways other than performing the most rudimentary of tasks, and they were able to conduct the first ever successful demonstration where a robot assisted resource nurses in the maternity ward of a hospital.
Rather than utilising robots to dispense medicine or to clean, the researchers wanted to find out whether it would be possible for a robot to serve as a "resource nurse" – a crucial role in maternity wards whereby one nurse is put in charge of delegating work to other nurses and making sure that all the patients are receiving the care they need and that procedures are being carried out in a timely manner with the resources available.
Hospitals are fast-paced, unpredictable environments with a great deal of complexity and clear decision-making can make the difference between life and death in emergency situations, and medical professionals have to be able to handle a great deal of pressure and still think clearly, while adequately managing their time and resources.
Teaching a robot about maternity care decisions
The researchers first trained a Nao robot to learn, with help from computer algorithms, how nurses routinely make scheduling decisions, such as which patient to be assigned to which room, and which nurse is required to perform a C-section and is available in case of an emergency during labour. The robot was taught to understand why exactly the scheduling nurses had chosen a specific decision rather than alternatives with the help of medical textbooks and papers.
Once the robot was able to grasp the complexity of the multitude of patients being assigned to various nurses as well as the nurses' availability and timelines on how labour progresses once patients arrive in a model that was similar to an actual hospital, Nao was then deployed to the partner hospital Beth Israel Deaconess Medical Center in Boston, US.
In one case, Nao was stationed with a resource nurse, who was in charge of coordinating 10 nurses, 20 patients and 20 rooms at the same time, but the robot was also tested with 16 other doctors and nurses.
Doctors and nurses followed the robot's advice 90% of the time
The participants were required to play a 10-minute-long video that simulated a total of four hours on the labour floor, factoring the patients that would require care and which nurses were available at the time to attend to them. During the simulation, the computer controlling Nao would interject and make recommendations about which nurse would be best placed to care for which patient, as well as advice on how rooms should be assigned.
Nao would deliver the advice verbally and then the participants would need to tap a button on the screen of the robot to accept or reject the advice, and the study found that doctors and nurses chose to follow the advice given by the robot 90% of the time.
"I think the [robot] would allow for a more even dispersion of the workload amongst the nurses. In some hospitals...more junior nurses were given the next patient...more senior nurses were allowed to only have one patient as opposed to two," one physician told the researcher when asked for feedback, while other nurses said that the robot would be able to help new nurses get to grips with the complex role of being a resource nurse much faster and could act as a good training tool.
The open-access paper, entitled "Robotic Assistance in Coordination of Patient Care" is available on the MIT CSAIL website.
© Copyright IBTimes 2025. All rights reserved.