New machine-learning satellite model can change how we gather data in space
University of Oxford's team trains a machine learning model on a satellite, enabling real-time monitoring and decision-making for remote-sensing satellites.
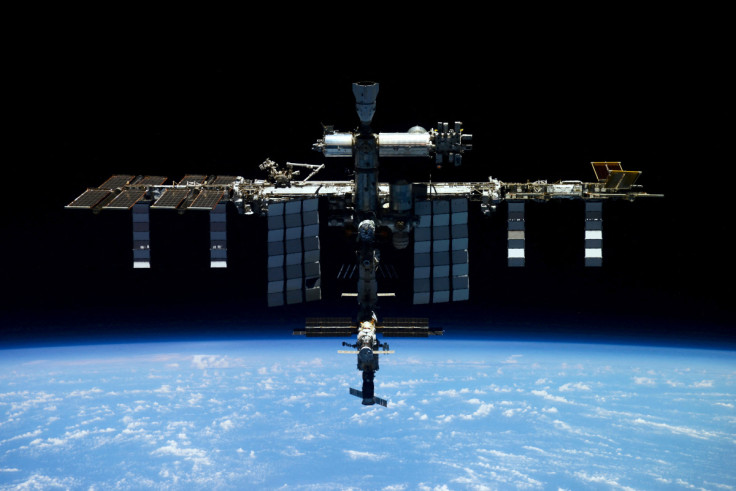
In an unprecedented achievement, researchers from the University of Oxford have successfully trained a machine learning model in outer space, on board a satellite, paving the way for revolutionary advancements in the capabilities of remote-sensing satellites.
Led by DPhil student Vít Růžička from the Department of Computer Science at Oxford, this groundbreaking project has the potential to enable real-time monitoring and decision-making for a myriad of applications.
The data collected by remote-sensing satellites has been a cornerstone for numerous crucial activities, including aerial mapping, weather prediction and deforestation monitoring. However, the current limitation lies in the passive nature of these satellites, which can only gather data and transmit it to Earth for processing. This process often takes hours or even days, hindering the timely identification and response to rapidly emerging events such as natural disasters.
In a bold initiative to overcome these constraints, the team led by Vít Růžička took on the challenge of training a machine learning programme directly in outer space. Their idea found its way onto the Dashing through the Stars mission, which had invited project proposals for implementation on the ION SCV004 satellite, launched in January 2022. During the autumn of the same year, the team successfully uploaded the programme's code onto the orbiting satellite.
The researchers focused on training a simple yet highly efficient model to detect cloud cover changes from aerial images right on the satellite itself. Unlike conventional approaches that involve ground-based training, the team utilised few-shot learning, a technique enabling the model to learn important features from only a few training samples. This compression of data into smaller representations made the model faster and more efficient.
Vít Růžička elaborated on their breakthrough, stating: "Our developed model, RaVAEn, compresses large image files into 128-number vectors. During the training phase, the model learns to keep only the informative values in this vector—those that relate to the change it is trying to detect, such as the presence or absence of clouds. This results in an incredibly swift training process due to the small classification model."
While the first part of the model, involving image compression was trained on the ground, the second part responsible for cloud detection was trained directly on the satellite. The team's tiny model achieved the remarkable feat of completing the training phase, using over 1,300 images, in a mere one and a half seconds.
Upon testing the model's performance on novel data, it demonstrated remarkable speed, automatically detecting the presence of clouds in approximately one-tenth of a second. The model's analysis covered an area equivalent to approximately 4.8x4.8 km² or nearly 450 football pitches.
The researchers are optimistic about the model's versatility, emphasising its potential for adaptation to various tasks and datasets.
Vít Růžička envisions: "Having achieved this demonstration, we now intend to develop more advanced models that can automatically differentiate between changes of interest, such as flooding, fires, and deforestation, and natural changes, like shifts in leaf colour across seasons. We also plan to explore complex data, including images from hyperspectral satellites, which could enable detecting methane leaks, holding crucial implications for combating climate change."
Furthermore, conducting machine learning in outer space may resolve the issue of satellite sensor calibration, affected by harsh environmental conditions. The proposed system could prove invaluable in constellations of non-homogeneous satellites, where reliable information from one satellite can calibrate the rest.
Vít Růžička explained: "This could recalibrate sensors that have degraded over time or experienced rapid changes in the environment."
The successful implementation of a machine learning model directly on a satellite marks a groundbreaking achievement with vast implications for space exploration and Earth monitoring. It opens new doors for real-time data analysis and decision-making capabilities, enabling us to respond swiftly and effectively to ever-changing global challenges.
As researchers from the University of Oxford continue to develop more advanced models, the future of spaceborne machine learning appears brighter than ever before.
© Copyright IBTimes 2024. All rights reserved.