Anticancer drugs breakthrough as neural network computers help create new medicines
Computer scientists prove artificial intelligence can drastically reduce time spent researching new drugs.
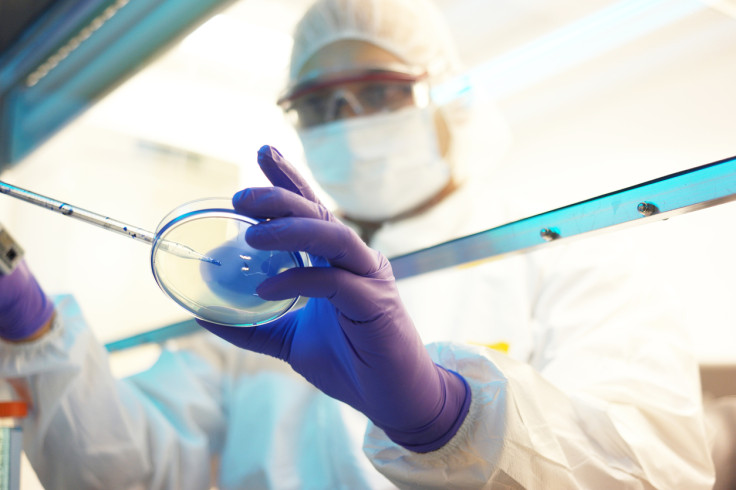
An international group of computer scientists have made a breakthrough that could totally revolutionise how anticancer drugs are developed – they have built a deep learning neural network that can research and discover new formulas for medications all by itself.
Researchers from the Moscow Institute of Physics and Technology (MIPT), Mail.Ru (Russia's Google), John Hopkins University, Kazan Federal University, the Russian Academy of Sciences, the University of Oxford and the UK-based Biogerontology Research Foundation have developed a neural network that is able to understand all the millions of organic chemical substances used in anticancer drug production and figure out how to combine the molecules to form new drugs.
Pharmaceutical research is incredibly difficult – there are hundreds of millions of substances in inorganic chemistry, but only a tiny fraction of these substances are used in medicinal drugs. A lot of drug research involves creating a compound and then continuing to repeatedly alter it ever so slightly in the laboratory to see if it will be better or safer for human use. As you can expect, this takes a very, very long time.
For example, the over-the-counter painkiller aspirin has been readily available and in use for over 100 years, yet its chemical compound acetylsalicylic acid continues to be researched by pharmacologists who hope to make it more efficient and reduce side effects.
Repeated failure in drug development is highly likely before positive results are achieved – in fact, clinical trials for all types of medication to treat diseases currently have a failure rate of almost 90%.
But what if you could get the computer to do some of that painstaking work for you? This is what the researchers have done, by developing a new technique that makes use of a type of deep learning called Generative Adversarial Networks (GAN). Developed in 2014, the GAN system enables unsupervised machine learning by getting two neural networks to compete against each other to improve the end result.
Training the neural network to recognise 72 million molecules
What are neural networks?
Neural networks are large networks of artificially intelligent classical computers that are trained using computer algorithms to solve complex problems in a similar way to the human central nervous system, whereby different layers examine different parts of the problem and combine to produce an answer.
In the last two years, computer scientists have demonstrated that neural networks can process images to produce surprising results, such as psychedelic paintings and amazing selfie filters, or use the images to learn to recognise and detect patterns, such as recognising blurred faces from photographs, or predicting social unrest several days in advance.
Using GAN, the researchers built a seven-layer neural network and trained the system to recognise the 72 million chemical compounds with known medicinal properties used in cancer chemotherapy as well as the exact concentrations required of each substance, using data from the PubChem database.
The Generative Adversarial Autoencoder (AAE) neural network consists of three parts – an encoder, a decoder and a discriminator, which all have a specific role and work together. When the researchers fed the information about the molecules to the neural network, the encoder and the decoder worked together to compress the information and then restore all the data belonging to each parent compound.
Meanwhile, the discriminator made the compressed data more suitable for being restored when the neural network produced the final result. Once the neural network learned all 72 million molecules, the encoder and the discriminator were turned off, and the network was able to generate a "fingerprint" for every single compound, containing complete information on it.
With knowledge of all the base compounds, the neural network then began to predict how the molecules should fit together to become anticancer drugs all by itself, to the extent that it predicted many existing anticancer drugs currently on the market, as well as brand new compounds that could work too.
Artificial intelligence can be used to discover new cancer drugs
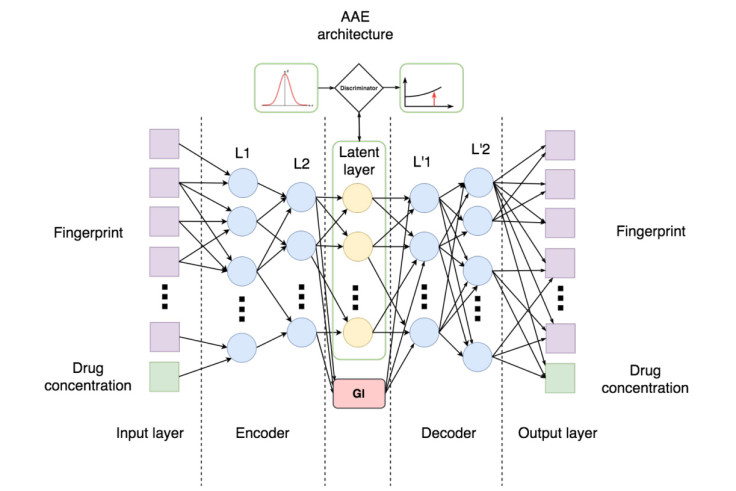
"While the use of deep learning methods in the biomedical field is still in its infancy and most of the applications are restricted to pure classification tasks, these techniques may transform drug discovery and biomarker development. In the present study, we demonstrated how DNNs can be used not only for classification tasks but also for the generation of biologically relevant models," the researchers concluded in their paper.
"The new conceptual architecture of AAE was used to develop and validate a complex deep learning-based workflow capable of generating models of new compounds in cancer and oncology using drug concentrations and fingerprints as sole inputs. As a result, we predicted the activity of 69 compounds belonging to various chemical classes.
"The anticancer activities for our prediction have already been identified and in some cases they are already used as anticancer agents for treating various cancer types including leukaemia and breast cancer. This confirms the ability of this approach to provide biologically relevant results. To the best of our knowledge this is the first application of GAN techniques within the field of cancer drug discovery."
Of course, we will not know if the new anticancer drug compounds predicted by the neural network will be potent, so the scientists suggest that these compounds could be statistically tested in future by the neural network using other computer algorithms, together with data about how cells have responded during experiments where human tissue is grafted into immunodeficient mice.
Their paper, entitled: "The cornucopia of meaningful leads: Applying deep adversarial autoencoders for new molecule development in oncology" is published in Oncotarget, a peer-reviewed open access medical journal dedicated to cancer research.
© Copyright IBTimes 2024. All rights reserved.