Why AI Alone Won't Sell: Chloé Portier on How Companies Can Monetise AI Effectively
AI's potential isn't enough - here's how businesses can turn innovation into sustainable revenue
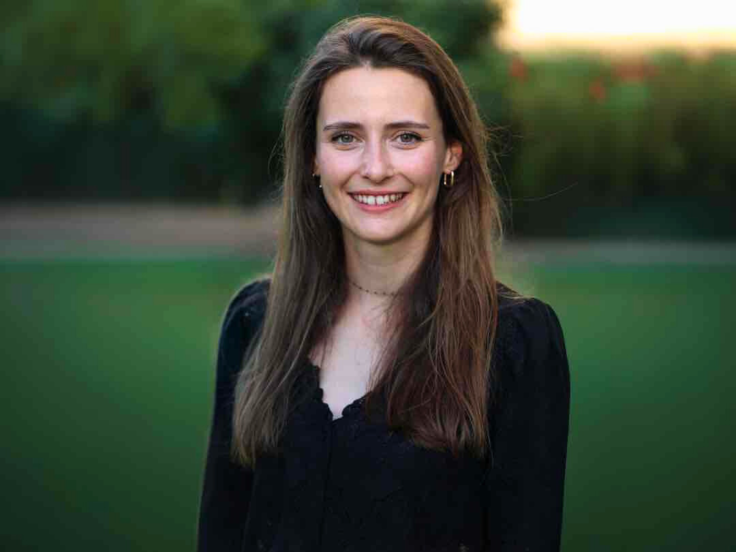
Multinational strategy and management consulting firm McKinsey estimates that more than 70% of businesses already use AI technology, with investments across industries expected to soar over the next decade.
However, the value equation has yet to be solved. As companies struggle to turn AI into reliable revenue streams, they may become less inclined to invest because of the high development and maintenance costs that come with the territory. AI has yet to prove itself to be a sustainable investment with positive long-term returns for many of them.
Few people understand this problem better than Chloé Portier, product manager and AI strategist at MadKudu, a company helping businesses improve their sales and revenue processes through AI-driven prospect insights. With a deep background in AI product development, she has worked extensively on commercialising AI-powered features—navigating the high cost of AI infrastructure, user adoption hurdles, and the complexity of value-based pricing to demonstrate AI's incredible impact on those who invest in it.
Who is Chloé Portier? The AI Monetization Expert Bridging Data and Business Strategy
Portier is a senior product manager and AI strategist at MadKudu, where she ensures that the company's AI solutions are commercially viable and offer tangible value to target clients at a realistic price point.
'I've always been drawn to solving problems with data,' says Portier, who earned two master's degrees in AI and data science in France before moving to San Francisco to specialise in business data science.
'That's where I realised I didn't just want to analyse data. I wanted to build the products that make AI useful.'
Portier noticed that basic business principles apply to AI as they do to any other business function. The best technology wouldn't work without a strong understanding of the user, and even the most technically impressive AI models would fail if they didn't solve a real need.
Her expertise in pricing, adoption strategies, and AI usability makes her one of the most insightful voices in AI monetisation. She works to consistently bridge the gap between data science and product, turning complex AI models into tools that help revenue teams make better decisions faster.
Portier has reviewed several major AI research conferences, including the Good Data Conference, IWHICI, GenAI4Health, and AI4EDU. She also co-hosts the AI Paper Bites podcast, where she breaks down complex AI research into digestible insights for industry professionals.
The Challenges of Monetizing AI: Hard Costs vs. Perceived Value
One of the biggest challenges in monetising AI-driven products is the vast gap between development costs and perceived value. 'It's slow, expensive, and hard to monetise,' she explains. The immense infrastructure costs are unlikely to be justified if the tool doesn't seamlessly integrate into user workflows.
'Buyers pay for outcomes, not the underlying complexity,' says Portier. 'If they don't immediately see the value, it's difficult to justify premium pricing—no matter how advanced the AI is.'
Amidst the current AI boom, it's tempting to assume that simply building AI features will generate more revenue. In truth, the expense of both building and running the technology often doesn't pay off. The companies that win aren't just the best at AI; they're the best at monetising it.
At MadKudu, Portier's team built an AI-driven copilot that analyses and contacts prospects based on LinkedIn profile details and key behavioural signals. While the tool had clear value, monetisation was challenging, raising questions about pricing for AI assistance and user trust. Instead of imposing an arbitrary pricing model, she prioritised adoption first, embedding AI into workflows, analysing user engagement, and demonstrating clear ROI before introducing a pricing strategy.
Once users began to see the tool's benefits, success metrics began to rise rapidly. The feature helped sales teams increase their pipeline conversion rates by 200% thanks to faster and more personalised outreach to potential prospects: 'My approach has always been adoption before monetisation. If a feature isn't solving a critical problem and driving habitual use, no pricing model will save it.'
Balancing AI's Development Costs With Sustainable Pricing
AI will likely always be expensive to develop. For Portier, this only increases the importance of building sustainable and profitable models.
'AI can automate a lot, but it shouldn't replace judgment,' she says. 'I've seen so many companies rush to 'AI-ify' their product without understanding if it improves user experience. The best AI-powered products don't just automate processes; they improve decision-making and remove friction for their users without removing control.'
Portier points to data network effects as a core means of making AI more economically successful. By continually collecting user data, AI features can drive more valuable insights, leading to greater adoption and more data in a virtuous cycle that builds efficiency over time.
'It's similar to what companies like Gong have done,' she explains. 'They've scaled their AI so that the marginal cost of delivering insights decreases as their data grows.' Over time, this lowered cost makes pricing models like per-seat pricing more sustainable, driving adoption.
On the other hand, Portier argues that consumption-based models can make sense for companies with high computing costs per user. Still, they risk slowing adoption because buyers become cost-conscious about usage. When pricing is tied directly to usage volume, customers become more cautious about how often they use AI features.
Instead, the best approaches to AI pricing strike a balance, delivering real value that solves a real need while keeping costs for both platforms and users in check to ensure broad adoption.
From AI Inflation to Sustainable ROI
Portier believes the AI industry is in a phase of 'AI inflation,' where companies overhype AI without proving its value. As trust declines, businesses must shift toward outcome-based pricing, tying AI to measurable revenue, efficiency, and retention improvements. While the technology is frequently hyped and showcased through new features, few companies can show its value.
Tying the technology to measurable revenue and efficiency improvement in this trying environment becomes more vital than ever. Chloé Portier is at the front line of that shift, using AI to accelerate product development cycles and enhance decision-making.
'I want to build AI-powered products that drive real-world impact,' Portier concludes. 'I want to create AI-driven solutions that optimise workflows and empower users by making complex tasks more intuitive and accessible.'
© Copyright IBTimes 2025. All rights reserved.