Artificial Intelligence May Help ADHD Diagnosis, Research Shows
The researchers said they discovered important differences in certain brain structures called white matter tracts in people with ADHD.
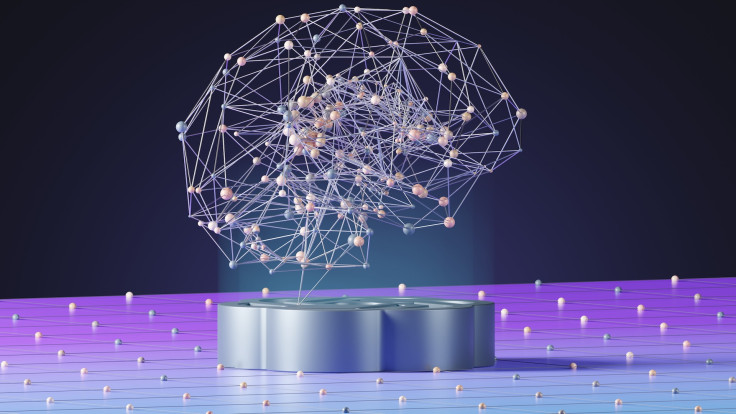
AI may help with attention deficit hyperactivity disorder (ADHD) diagnosis, new research shows.
ADHD is defined as being one of the most common neurodevelopmental disorders of childhood. It can cause difficulty in maintaining attention, managing energy levels, and controlling impulses.
Typically showing up in childhood, it can significantly affect an individual's well-being and their ability to function in society.
In the United States, approximately six million children and teenagers aged six to 17 have received a diagnosis of ADHD.
But up until now, diagnosis of the condition has often been unreliable, expensive and inconclusive.
With medical professionals often relying on self-reported surveys that are subjective, there is a clear demand for more objective methods of diagnosis.
In new research presented at the Radiological Society of North America's annual meeting, scientists reported on a deep learning type of AI to examine MRI scans of teenagers with and without ADHD.
Deep learning is a type of AI that can automatically recognize patterns and connections within vast amounts of data.
The researchers said they discovered important differences in certain brain structures called white matter tracts in people with ADHD.
The study, which hasn't been published yet in a peer-reviewed journal, is important because it's the first to utilize deep learning to identify indicators of ADHD.
Justin Huynh, MS, a study co-author and a researcher in the Department of Neuroradiology at the University of California San Francisco and a medical student at the Carle Illinois College of Medicine at Urbana-Champaign in Illinois, says that they analyzed a large dataset of brain images of adolescents with and without ADHD.
"We found that, on average, there were statistically significant differences in imaging between study participants with and without [attention deficit]," he said.
The research involved data from brain scans, clinical surveys, and other information gathered from 21 research sites in the United States.
Previous attempts to utilize AI for ADHD detection have faced difficulties due to small sample sizes and the intricate nature of the disorder, the researchers reported.
In this study, the research team specifically chose 1,704 individuals consisting of both adolescents with ADHD and those without the condition.
This research represents the latest example of AI's capability to transform medical diagnosis and treatment.
Last week, a groundbreaking study, in the esteemed journal Cancer Discovery, introduced a sophisticated AI algorithm capable of deciphering the intricate interplay of genetic mutations within tumours, revolutionising our ability to anticipate how cancer will respond to treatment.
The discovery comes under a year since pharmaceutical company AstraZeneca announced they will partner with US-based AI biologics firm, Absci, to design an antibody for cancer.
The collaboration aimed to create a zero-shot generative AI model, which would work on creating new antibody therapeutics and enhancing the existing ones.
Since then, there have been several breakthroughs harnessing the power of AI to aid the treatment of diseases.
Investigators at Harvard Medical School and the University of Copenhagen, for example, have developed an AI-based population screening that can expedite the diagnosis of pancreatic cancer. This groundbreaking tool promises to revolutionise cancer diagnosis and treatment, offering patients a faster, more accurate diagnosis and a better chance of survival.
In a report published this month, the World Health Organisation outlined the benefits and risks of AI use in the healthcare industry.
They identified five broad areas where the technology could be applied.
These are diagnosis, such as responding to patients' written queries; scientific research and drug development; medical and nursing education; clerical tasks; and patient-guided use, such as investigating symptoms.
But while this holds potential, WHO warned there were documented risks that LMMs could produce false, inaccurate, biased or incomplete outcomes.
They might also be trained on poor-quality data, or data containing biases relating to race, ethnicity, ancestry, sex, gender identity or age.
© Copyright IBTimes 2024. All rights reserved.