Deep learning and data science demand prompts portfolio managers to take night classes
NVIDIA will be offering a day-long training session on 5 December, as part of Newsweek's data science and capital markets event in New York next week.
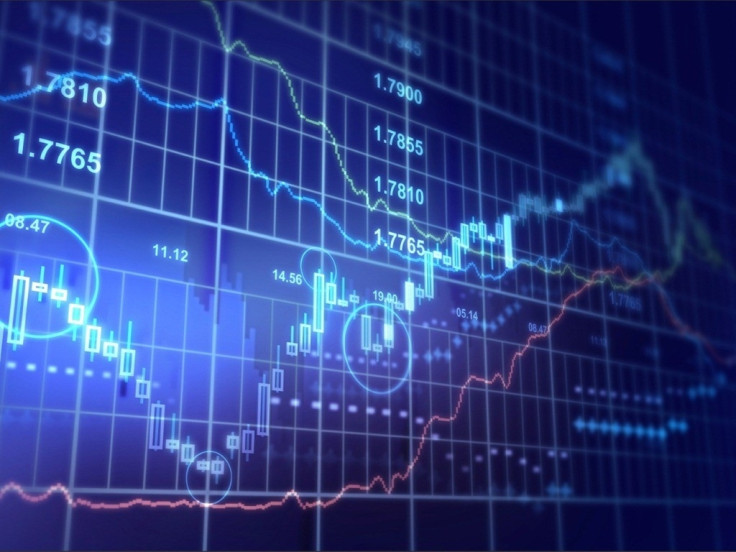
The demand for data science talent in the capital markets space has seen portfolio managers and discretionary traders attending night classes in a bid to safeguard their jobs amid a rising tide of automation.
The whole asset management industry is moving in the direction of being more systematic, being more quantitative and using new unique data. A lot of firms are struggling in many ways to get their heads around that. While there may be many vanilla data science training courses out there, finding a solid introduction for quantitative finance training with the nuanced level of applicability is not so easy.
Leigh Drogen, the founder of crowdsourced financial analysis platform Estimize, designed and created the L2Q (Learn to Quant) programme as a rudimentary introduction for discretionary managers.
Drogen said the specific questions at an introductory level include crucial things like how to get the most out of analysts in a structured way, or how to set up the firm to do quantitative research and feed that into a discretionary trading process.
He said: "They need to build a more systematic process to evaluate things: how to analyse new unique datasets; how do I become more factor aware.
"Part of the L2Q programme is about helping your firm build a process so the work you're doing can actually be integrated into the PM's book, because that's a really tough thing that they are all going through right now."
Estimize's training day includes lectures from the likes of WorldQuant and experts from independent research shops like Yin Luo at Wolfe, as well as quantitative analysts from MSCI.
"Firms like that that can teach the individual modules of factor modelling, data science and quant research; for example, how do you work with vendors; how do you know if a dataset is legitimate; how do you integrate it?
"I think it's a valuable thing for the discretionary PMs, analysts, COOs, capital allocators. We make sure that they know this is going to be a relatively rudimentary thing: it's the basics, but it's incredibly important," said Drogen.
For those initiated into the world of data science, high performance computing hardware firm NVIDIA recently launched its Deep Learning Institute (DLI) which offers a one-day introduction to its deep learning frameworks.
NVIDIA set out to develop labs that show how to easily marry the basic building blocks of deep learning like auto-encoders, recurrent neural networks, reinforcement learning, with finance problems like algorithmic trading, statistical arbitrage, optimising trade execution etc.
NVIDIA's GPU architecture is well-suited to algorithms that need to scale across many parallel calculations; its deep learning frameworks help deal with the diversity of networks.
Andy Steinbach, head of AI in financial services and senior director at NVIDIA, said: "Recurrent neural networks, for example, are good for financial engineering because they allow you to incorporate time series. Tools like auto-encoders help you get around the fact that you might not have labelled data, you might not actually know what you're looking for - some behavioural pattern - but you can't label it, you want the network to discover it.
"Deep learning frameworks allow researchers to very quickly structure these networks and not have to recreate the wheel in terms of software every time."
"It's interesting that most of these algorithms reduce to a master training algorithm under the hood, called back-propagation, which is highly parallel and makes it easier to scale the computations across many GPUs.
"That algorithm takes large streams of data – it can be images, it can be audio, it can be tick data – and trains these networks and all the parameters, and so these complicated deep learning algorithms map onto this relatively simple algorithm that's massively parallel.
"And if your deep neural network isn't training fast enough on a big data set, you can essentially push a button and scale it out to more GPUs in a data centre," he said.
NVIDIA will be offering a day-long training event on the 5 December, as part of Newsweek's data science and capital markets event in New York next week.