University of California Scientists Unleashes AI to Predict Chemotherapy Resistance in Cancer
The study, led by Dr Trey Ideker, a professor in the Department of Medicine at UC San Diego School of Medicine, showcases the potential of artificial intelligence in unravelling the complexities of cancer drug responses.
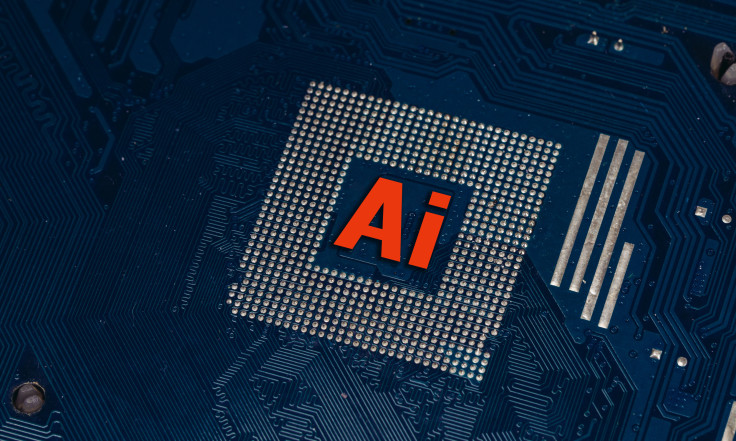
In a massive leap forward for cancer research, scientists at the University of California San Diego School of Medicine have harnessed the power of machine learning to confront one of the most daunting challenges in the field — predicting resistance to chemotherapy.
The groundbreaking study, unveiled on January 18, 2024, in the esteemed journal Cancer Discovery, introduces a sophisticated algorithm capable of deciphering the intricate interplay of genetic mutations within tumours, revolutionising our ability to anticipate how cancer will respond to treatment.
Cancer cells, like all cells, rely on intricate molecular machinery to replicate DNA during cell division. Chemotherapies typically disrupt this DNA replication process, particularly in rapidly dividing tumour cells.
However, the unpredictable nature of the vast array of mutations found within tumours has long confounded researchers attempting to predict drug resistance.
The newly developed machine learning algorithm transcends this challenge by analysing how numerous genetic mutations collectively influence a tumour's response to drugs inhibiting DNA replication.
The researchers focused their efforts on cervical cancer tumours, utilizing their model to predict responses to cisplatin, a widely used chemotherapy drug. The algorithm not only identified tumours most susceptible to treatment resistance but also illuminated the underlying molecular machinery driving this resistance.
Trey Ideker, PhD, a professor in the Department of Medicine at UC San Diego School of Medicine, explained the significance of the study, saying: "Clinicians were previously aware of a few individual mutations that are associated with treatment resistance, but these isolated mutations tended to lack significant predictive value.
"The reason is that a much larger number of mutations can shape a tumour's treatment response than previously appreciated."
Artificial intelligence, in this instance, acts as a bridge to understanding this complexity, allowing for the simultaneous analysis of thousands of mutations.
Ideker noted: "Rather than focusing on a single gene or protein, our model evaluates the broader biochemical networks vital for cancer survival."
The inherent complexity of DNA replication, involving hundreds of proteins working together, adds another layer of challenge in comprehending how tumours respond to drugs. Mutations in any part of this intricate system can alter the tumour's response to chemotherapy.
The researchers utilized a standard set of 718 genes commonly employed in clinical genetic testing for cancer classification. These genes provided the initial input for their machine-learning model, which, after training with publicly accessible drug response data, identified 41 molecular assemblies or groups of collaborating proteins. These assemblies proved to be crucial in understanding how genetic alterations influence drug efficacy.
"Cancer is a network-based disease driven by many interconnected components, but previous machine learning models for predicting treatment resistance don't always reflect this," Ideker said. "Our model evaluates the broader biochemical networks vital for cancer survival."
Putting their model to the test in cervical cancer, where approximately 35 per cent of tumours persist after treatment, the researchers found it to be adept at identifying tumours susceptible to therapy, correlating with improved patient outcomes. The model also accurately pinpointed tumours likely to resist treatment.
Moreover, the model provided insights into its decision-making process, shedding light on the protein assemblies influencing treatment resistance in cervical cancer.
Ideker stressed the importance of the model's transparency, saying: "Unravelling an AI model's decision-making process is crucial, sometimes as important as the prediction itself."
The researchers believe that the transparency of the model is a key factor in its success and essential for building trustworthy AI systems.
Ideker explained: "Our model's transparency is one of its strengths, first because it builds trust in the model, and second because each of these molecular assemblies we've identified becomes a potential new target for chemotherapy.
"We're optimistic that our model will have broad applications in not only enhancing current cancer treatment but also in pioneering new ones."
© Copyright IBTimes 2024. All rights reserved.