Can machine learning be applied to the problem of trading?
NYU professor Gordon Ritter constructed an artificial system which he knew would admit a profitable strategy, to see if a machine would find it.
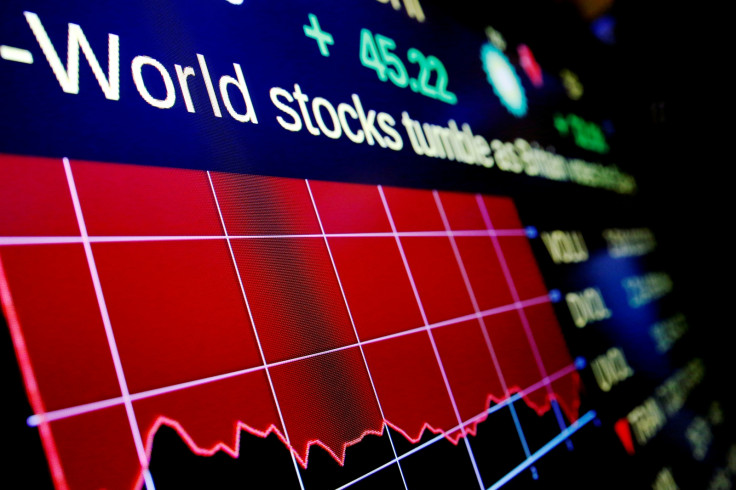
A new academic paper, Machine Learning for Trading, is the first conclusive study that shows success from having a machine learning-based trading strategy.
The author, Gordon Ritter, Adjunct Professor in the Mathematics in Finance Program, New York University, constructed an artificial system which he knew would admit a profitable strategy, to see if a machine would find it.
In order to train a machine learning algorithm to behave as a rational risk-averse investor required appropriate reinforcement learning, specifically a mathematical technique called Q-learning (playing some sort of game where you are trying to maximise the reward function that may occur at several periods in the future).
The machine learning agent found and exploited arbitrage opportunities in the presence of transaction costs in a simulated market proof of concept.
Ritter explained: "I was really trying to answer the question, does machine learning have any application to trading at all, or no application; sort of a binary question. Can machine learning be applied to the problem of trading?
"I reasoned that in a system that I know admits a profitable trading strategy, because I constructed it that way, can the machine find it. If the answer to that is no, then what chance would it have in the real world where you don't even necessarily know that a profitable strategy exists in the space you are looking at.
"So luckily the answer to that was yes. In the system where I knew there was a profitable opportunity, the machine did learn to find it. So that then allows for further study."
It's not uncommon to hear sophisticated quant shops espousing the opportunities of machine learning, but the fact remains that these firms don't really publish anything about how well this works.
"To my knowledge there has been no conclusive study that shows any success in having a machine learning-based trading strategy. I believe this paper to be the first," said Ritter.
"From the standpoint of academic research published in peer review journals, there aren't a lot of studies of the application of machine learning to trading - yet. But I think there will be."
As mentioned, Q-learning is a way of solving a kind of delayed reward problem where you may have to think several moves in advance. Anyone who has played chess, Go, or even Pac-Man will be familiar with the idea that you have to think of what's going to happen in several time periods, and not just the very next time period.
Ritter said: "You can't be myopic and win these games. So reinforcement learning is a general framework for solving those types of problems where you have a delayed reward or you are trying to maximise a cumulative reward over time.
"In trading the same sort of thing occurs. You might build up a position with the expectation of a long term gain on that position and a short term loss is also possible, but in the anticipation of a longer term gain you might be willing to make that trade."
Going back to the 1950s, the economic theory of portfolio choice and utility maximisation has included various approaches to the concept of risk; portfolios are optimised not just for expected gain, but expected gain minus a penalty for risk.
"When setting up reinforcement learning, you have to have some sort of score," said Ritter. "If it's a game, like a video game it might literally be the score in the game. But generally speaking the score could be a more abstract notion; it could be anything that you are trying to maximise over time. In portfolio choice it's not a new concept, what you really want to maximise over time is gain minus a penalty for risk."
A question on many people's lips when it comes to machine learning and AI-driven automation concerns the role that will be left for humans.
Ritter takes a sober view of this: "I think it's important to know what human beings are good at and what they are not good at. So human beings are typically not good at knowing what their true costs are.
"For example, we all know that doing a large trade or a trade that's a large fraction of the volume can have an impact in the market; you'll move the price as a result of your trading. Well, how much?
"Humans are not really good at answering that question. That's a better question for a mathematical model. So we sometimes get asked the question, does this mean humans are done? I think the answer to that is definitely not.
"Humans are probably good at coming up with the idea for a new strategy. Take the technology in my paper, for example: where should we apply it? What should we apply it to? What should the signal that drives the trade be, if any?
"But humans are not good at interacting with the microstructure; humans are not good at looking at a bid and an offer and saying, I think if I execute this many basis points here's what my impact will be. That kind of decision should be left to a machine really."
Gordon Ritter will be talking about machine learning at Newsweek's AI and Data Science in Capital Markets conference on December 6-7 in New York, the most important gathering of experts in Artificial Intelligence and Machine Learning in trading. Join us for two days of talks, workshops and networking sessions with key industry players.